Enhancing Business Security with Machine Learning Spam Detection
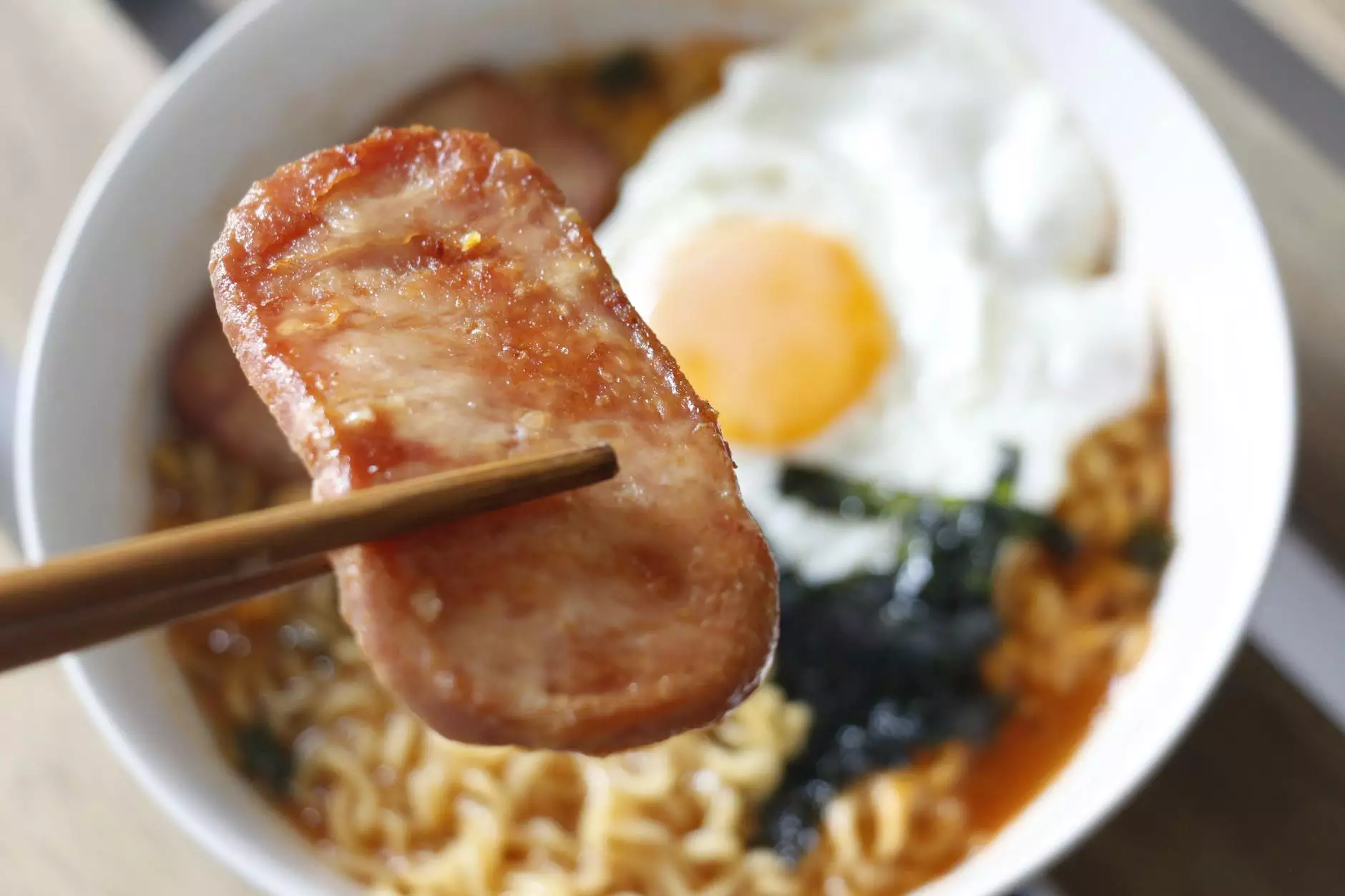
The ever-evolving landscape of digital communication presents unique challenges for businesses today. Spam emails and malicious content can undermine productivity and pose significant security risks. In this article, we will delve into the transformative power of machine learning spam detection, explaining its benefits, functionality, and its critical role in IT services and security systems. We will explore how businesses can leverage this cutting-edge technology to enhance their operations and protect their digital assets.
The Necessity of Spam Detection in Modern Business
With the increase in email communication, spam attacks have become more sophisticated. Statistics show that over 50% of all emails sent globally are classified as spam. For businesses, this not only leads to wasted time and resources but can also result in security breaches. The need for robust spam detection mechanisms has never been more pressing. Here’s why:
- Time Wastage: Employees spend significant time sorting through spam emails, which distracts from core tasks.
- Security Risks: Spam emails are often a carrier for phishing attempts and malware.
- Reputation Management: Failing to prevent spam can lead to a business's email reputation being compromised, which affects communication and trust.
What is Machine Learning Spam Detection?
Machine learning spam detection is a sophisticated technology that utilizes algorithms to identify and filter out spam emails. Unlike traditional spam detection methods, which rely primarily on pre-defined rules and keyword matching, machine learning employs data-driven approaches that adapt and improve over time. Specifically, it includes:
- Algorithm Training: Machine learning algorithms are trained on large datasets, learning to distinguish between spam and legitimate emails.
- Pattern Recognition: These algorithms continuously recognize patterns in emails, allowing for real-time adaptations to emerging spam techniques.
- Feedback Loop: User inputs help refine the algorithms further, as they learn from both false positives and false negatives.
How Does Machine Learning Enhance Spam Detection?
Machine learning enhances spam detection through advanced techniques that significantly improve accuracy and efficiency. Here are some key methodologies:
1. Classification Algorithms
Machine learning uses various classification algorithms, such as:Naive Bayes, Support Vector Machines (SVM), and Random Forests, to effectively categorize emails as spam or not. By analyzing numerous features—such as sender reputation, email content, and metadata—these algorithms achieve high levels of precision.
2. Natural Language Processing (NLP)
NLP allows for a deeper understanding of the context within emails. Instead of simply flagging based on specific keywords, machine learning models can analyze the text and determine whether the tone and context signify spam.
3. User Behavior Analysis
Machine learning models can learn from user behavior by analyzing how individuals interact with their emails. Patterns can indicate legitimate messaging habits, enabling better recognition of potential spam.
4. Real-time Learning
One of the most significant advantages of machine learning spam detection is its ability to adapt based on real-time data. As new spam techniques arise, the system can learn from these trends instantly, ensuring users are always protected.
Benefits of Machine Learning Spam Detection for Businesses
Implementing machine learning spam detection in businesses comes with a myriad of benefits:
1. Enhanced Security
By filtering out spam effectively, businesses minimize the risk of security breaches that can compromise sensitive information. This enhanced protection is essential in today’s digital landscape.
2. Improved Productivity
Employees spend less time dealing with spam emails, allowing them to focus on productive tasks that drive business success. This leads to improved efficiency across the board.
3. Cost-Effective Solutions
Reducing the amount of spam can decrease the need for extensive IT support to manage security incidents. This streamlining of operations translates to significant cost savings.
4. Better Customer Engagement
When legitimate communication is not drowned out by spam, businesses can better engage with customers and their needs, leading to improved satisfaction and loyalty.
Implementing Machine Learning Spam Detection in Your Business
For businesses looking to enhance their security through machine learning spam detection, several steps can be undertaken:
1. Choosing the Right Tools
Select a machine learning tool or service that fits your business needs. Options can range from integrating third-party solutions to building custom models based on specific requirements.
2. Data Collection
Effective machine learning depends on quality data. Businesses should ensure they have access to a comprehensive dataset of emails, including examples of both spam and legitimate messages.
3. Continuous Training and Evaluation
The effectiveness of spam detection systems greatly depends on continuous learning. Businesses should engage in regular evaluations of their spam detection systems and retrain models with new data.
4. User Training
Provide training to employees on recognizing and handling spam appropriately, including how to report false positives or negatives. This feedback loop can help improve the overall accuracy of the system.
Case Studies: Success Stories in Industry
To illustrate the robust effectiveness of machine learning spam detection, here are a few brief case studies from various industries:
1. E-commerce
One leading e-commerce platform adopted a machine learning spam detection system, resulting in a 90% reduction in spam reaching user inboxes. This shift significantly improved user trust and engagement rates.
2. Financial Services
A multinational financial institution employed an AI-driven spam filter, enhancing their security framework. They reported that phishing attempts decreased by over 70%, protecting sensitive customer data while maintaining compliance with regulations.
3. Healthcare
A healthcare provider implemented machine learning spam detection and saw a marked improvement in communication efficiency. Healthcare professionals experienced a 60% decrease in spam, allowing them to focus more on patient care.
Future Trends in Machine Learning Spam Detection
The landscape of spam detection is continually advancing, and future trends indicate even greater integration of machine learning with other technologies, including:
- Predictive Analytics: Utilizing predictive techniques to anticipate spam trends based on historical data.
- Integration with Other Cybersecurity Measures: Combining spam detection with endpoint security solutions for holistic protection.
- Improving User Experience: Focusing on minimizing false positives to ensure legitimate emails are not flagged.
Conclusion
In conclusion, machine learning spam detection is a transformative solution for businesses aiming to enhance their security frameworks and streamline operations. By utilizing cutting-edge technology, companies can fend off spam effectively, thereby boosting productivity and securing sensitive information. As the threats of spam continue to evolve, so too must the approaches to tackling them. By adopting machine learning as a foundational element in spam detection strategies, organizations not only safeguard their operations today but also prepare for a more secure digital future.
For businesses ready to take that proactive step, Spambrella offers a range of solutions in IT Services & Computer Repair and Security Systems tailored to meet the unique needs of every enterprise. Embrace the power of machine learning spam detection today and secure your business’s place in an increasingly complex digital ecosystem.